AI Success In The Supply Chain Depends On Human Augmentation
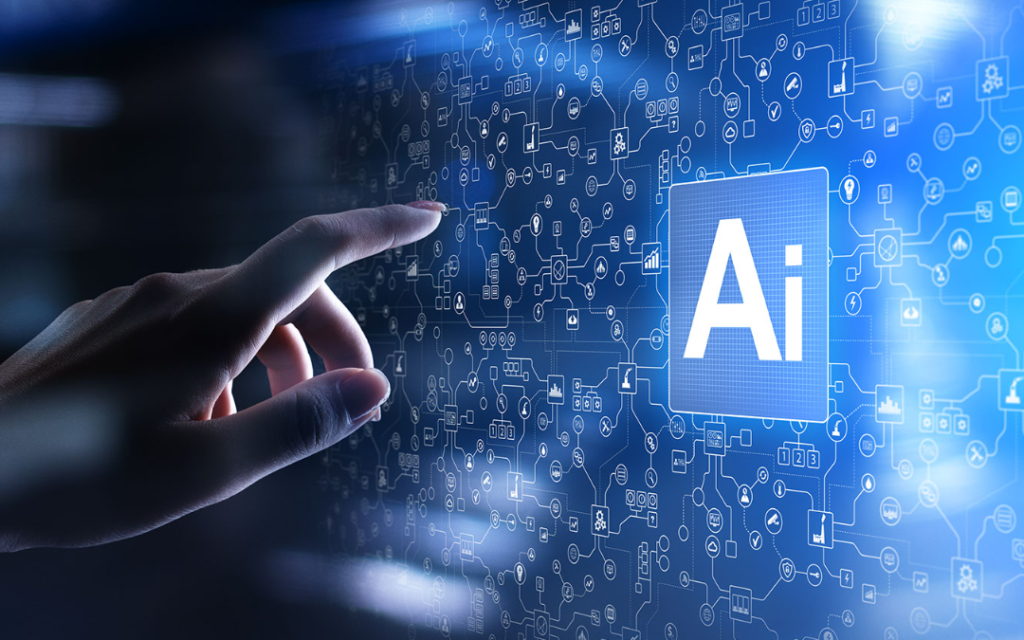
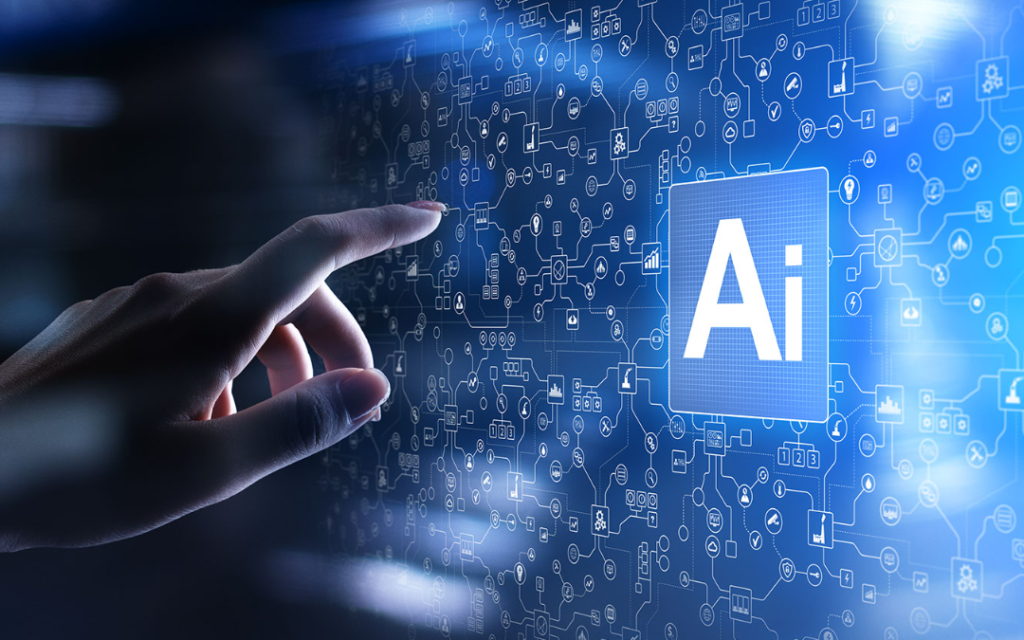
The past year showed how vital the continuity of the supply chain is to healthcare, goods manufacturing, and practically every facet of everyday life. The recent attention the industry has experienced, (as shortages continue to mount), has also led to a massive wake-up call for the supply chain to closely embrace digital transformation. Within this thread of having a more precise and actionable view of inventory, AI is playing a critical role in accelerating the supply chain. AI in the supply chain management market is expected to reach $1.3 billion by 2024.
But AI success within the supply chain can’t happen without real life human augmentation. You can’t just plug in IBM’s Watson and expect it to know everything about your company. Watson was programmed—or taught by a human — how to play chess and answer trivia questions, just like AI must be instructed for its role within your organization’s supply chain. Here are three ways that human augmentation determines the successful utilization of AI.
Knowledge Transfer
Organizations using legacy systems are reliant on human interaction. Legacy systems are rigid when it comes to the decision-making processes, not taking into account the changes that happen along the line—from extreme changes in consumer buying patterns to adjustments in stock supplies.
Another important aspect of knowledge transfer is harnessing the expertise of an experienced but also aging workforce. Yes, machines will replace some workers who are retiring or moving on to other positions, but robots can’t replace the vast amount of experience and knowledge that will leave with these employees.
Think of knowledge transfer like teaching information to a toddler. If a toddler is taught bad habits, that’s how they’ll model their behavior going forward. If AI is taught bad habits, it will produce wrong decisions and negative outcomes, which will adversely impact the organization and its supply chain. Humans have to guide AI on the right path, transferring knowledge and learnings built from rich past experiences.
Purpose-Built
When AI is introduced into the supply chain, it is done so for a specific purpose. However, AI doesn’t automatically know what its role is. Its purpose must be built into the model.
For example, an AI model that is supposed to route trucks to the right place won’t work properly unless it knows what parts are needed at the final destination. AI can’t make decisions it hasn’t been taught how to make. Humans are responsible for teaching the machine the correct information. When AI is given the wrong information, it is up to human intervention to discover and fix the algorithms, driving perfection in outcomes. For example, only experienced employees will know the patterns of the company’s e-commerce site and the number of raw materials needed at specific times of the year to meet demand. That expertise is needed to have an AI model present the information for a decision to be confirmed and to have it learn.
The importance of how data is programmed into AI models can’t be stressed enough. Humans may know that “bearing” and “BRG” mean the same thing within supply chain materials or that “five inches by ten inches” equal 5” by 10”, but AI won’t know this unless it is built to recognize that two things can have the same meaning via Natural Language Processing (NLP).
A Unique Human Augmentation Solution
An innovative way to accomplish accelerated AI programming is through collaboration with human augmentation companies. Here at Verusen, we are working with Daivergent, a company that helps unlock the unique aptitude of people on the autism spectrum, connecting companies to a high-quality remote workforce. Daivergent employees are helping Verusen tackle the seemingly impossible challenges related to further developing AI and machine learning specifically as it is related to solving supply chain issues.
Verusen and Daivergent’s collaboration pairs people with specific job types and tech problem solving related to targeted AI data training that improves outcomes. Specifically, Daivergent employees provide Verusen with annotations, training data, for both new and exploratory AI tasks. For example, they have provided training data for identifying similar materials across data sets, abbreviation expansion (e.x. expand the part description: “BRG RLLR CY321CR KOYO” —> “BEARING ROLLER CY321CR KOYO”), and manufacturer equivalency. Such annotations are being used to provide examples to existing and next-gen Verusen AI supply chain systems and our AI and machine learning platform.
Human Execution
Your organization could have the coolest new AI system, with all sorts of bells and whistles that offer new ways to streamline the supply chain, but if your AI models aren’t executed properly, it will have little to no positive outcome or impact and run the risk of losing trust from its human partners.
What is meant by execution? Execution starts by transferring knowledge and getting validation that AI has the right information. The AI must be purpose-built with a distinct goal. AI is going to analyze this information into useful data. Now it is up to the human to take what the AI has told them and put it into action. For example, an organization builds a model to look at the pain points in the supply chain, and after analysis, it is verified that you can save $30 million by making specific changes. While AI can handle some of those functions, it is up to humans to work across departments and execute them — from operations to logistics to finance. This is critical as companies embrace digital transformation, where each area is working in its silo. It is only through human augmentation and understanding how everything works together that AI success in the supply chain will happen.