Revolutionizing Materials Inventory Data
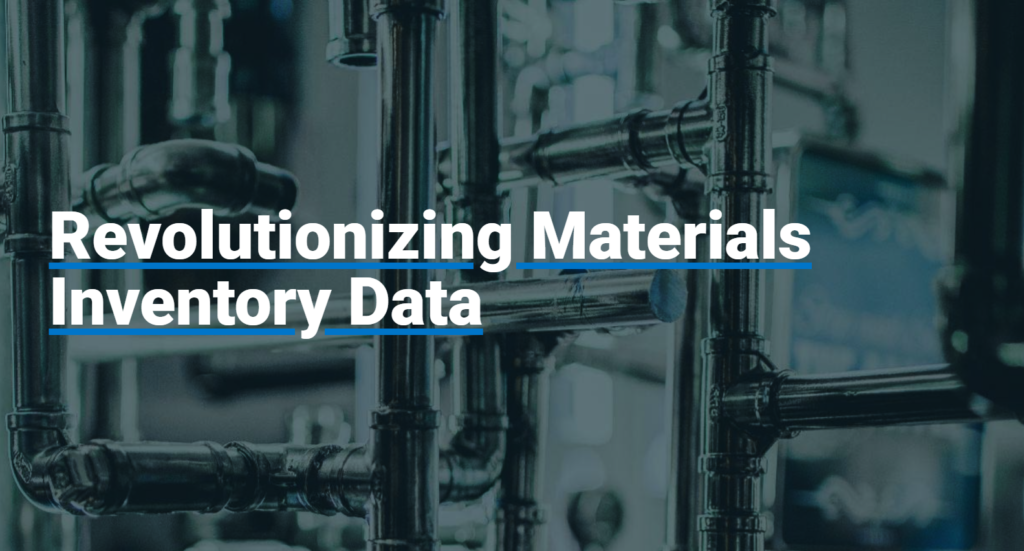
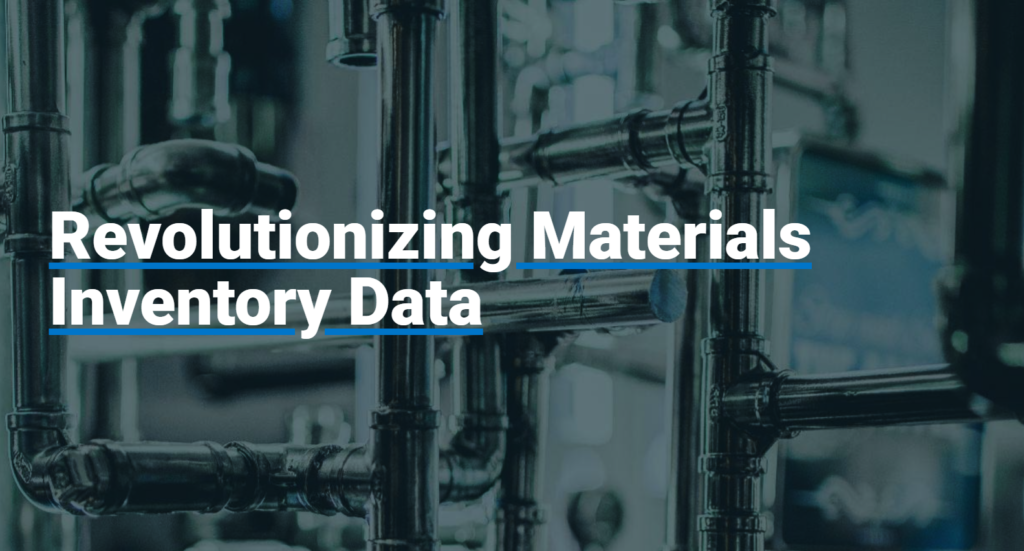
AI as the Answer to Greater Visibility and Lower Supply Chain Costs
In today’s demanding business environment, accurate management of materials inventory is a necessity for optimal business agility and financial performance, yet inventory data is often unreliable. Disorganized, disparate and inaccessible data has many causes and results in increased supply chain costs. Bad data also leads to inaccurate and unpredictable inventory forecasts that result in an ever-growing inventory of obsolete, slow-moving or overstocked items. Perhaps more importantly, lack of parts and resulting manufacturing downtime can jeopardize product deliveries and customer relationships — and ultimately result in loss of revenue.
The average Fortune 500 manufacturer has anywhere from $40-$60 million in wasted working capital tied up in excess parts (and up to $100 million for just four weeks of inventory in some industries¹). For enterprises that rely on data to propel their inventory optimization and other supply chain initiatives, bad data can be especially damaging to the bottom line.
Luckily, we’re at the outset of the Fourth Industrial Revolution,² Industry 4.0³ — as well as Supply Chain 4.0,⁴ where advanced digital technologies like artificial intelligence (AI) are applied to operational processes. More specifically, AI’s integration with enterprise resource planning (ERP) solutions—the most common custodian of materials inventory data—is predicted to profoundly transform the ERP footprint and allow organizations to optimize their business processes and drive down operational costs,⁵ such as those from inaccurate materials data.
The use of AI for materials inventory optimization offers companies a way to manage inventory, costs and uptime while eliminating the manual aspect of resolving real inventory visibility. Understanding the causes of bad materials data and learning how to correct them helps “harmonize” inventory systems data to provide a more accurate, reliable and reportable materials master that supports an agile supply chain and improved financial performance.
Common Culprits in the Data Accuracy Struggle
The very data backbones of enterprises — their ERP systems — are often the chief offenders when it comes to bad data, though inadvertently. ERPs create confusion as numerous employees enter data and extract information from centralized sources or custom modules, and this personalized use of the various ERP modules results in different understandings of the platform. For example, the procurement function will use ERP data much differently than plant maintenance staff.
Also, for many organizations, their ERPs are licensed and stored on-premise, disconnecting them from system upgrades and the ability to leverage the latest technological advancements. Especially in cases where companies grow through acquisition, legacy systems like ERPs continue to pile on top of each other, adding to the disparate data.
While the efficiency of inventory data management is a product of the defined capabilities of a given ERP system, the human factor plays heavily — starting with data input. For example, many material masters in ERPs provide character-limited free text fields for material descriptions. Without clear data management practices or a system that appropriately guides the user, employees often input the new materials data they value without considering how other colleagues, divisions or facilities reference and search for that same item. In addition, searching for material availability is difficult because ERPs require strict syntax and can be thrown off by typing errors or naming conventions (e.g.., searching 120 horsepower vs. 120HP). Either issue can result in costly and inaccurate inventory visibility that threatens supply chain efficiency.
The Bad Data Domino Effect
With or without the presence of ERP systems, the negative impacts of duplicate and inaccurate inventory data cascade down through the enterprise. This is especially true for the manufacturing plant and procurement function.
Downtime in the Manufacturing Plant
Having inaccurate materials inventory data means you are gambling with unplanned downtime, production efficiency and the financial bottom line. For example, a survey of automotive industry executives placed the average cost of stopped production at $22,000 per minute.⁶
When technicians can’t quickly locate a part, they may have extended periods of downtime for manufacturing equipment. The part may actually be in stock but was never located because of poor inventory naming conventions, descriptions, etc. The unplanned downtime costs the company significantly from a production perspective and could potentially threaten customer relationships. On the flip side, well-managed materials inventory could lead to greater service levels for customers that result in additional orders.
Also, concerns about unplanned downtime often lead plant buyers to hold more inventory to mitigate lack of materials. Without good visibility of the materials inventory data and an understanding of what’s truly on-hand, the “insurance” stock tends to be at a much higher level than necessary, increasing costs.
Procurement
The procurement function helps the business strategically buy materials and control spending. When someone can’t find a part, the plant submits procurement requisitions for the seemingly out-of-stock materials, despite actually having it under a different material ID and description. New materials are then ordered, leading to overstocked inventory and higher working capital expenditures.
Also, if the search-and-locate process is cumbersome and time-intensive, staff may directly order parts on a procurement card (“p-card”). Not only is excess inventory being added, but purchases are made without the transparency that enables procurement to leverage spend and run strategic sourcing events for better pricing as an enterprise.
The cost of excess inventory varies by industry and can be significant. For example, a heavy equipment manufacturer with expensive components might keep four weeks of inventory valued at $100 million.¹ If it could confidently cut that inventory to three weeks, it would save $65 million in just one year.¹
In a recent survey of medium-to-large manufacturing firms, nearly all responding executives believed that a data-driven procurement process is vital to achieving long-term value, cost reductions and efficiency gains.⁷ In a survey of chief procurement officers, 17 percent said they had no digital procurement strategy, and of those that did, less than one-third believed their strategy would help deliver on procurement objectives.⁸ The fact remains, however, that regardless of an organization’s state of digital procurement, the technologies adopted will only be as good as the underlying data.
Common and Costly Data Cleansing Tactics
Internal MDM, IS and Structured Processes
From an internal standpoint, the Master Data Management (MDM) or Information Systems (IS) teams are charged with keeping the data clean and free of redundancies, while providing guidance for strategic data opportunities. These teams are on the front lines of managing bad data, so they’re spending increasing amounts of time, effort and money on what is a laborious manual process that, in most cases, involves serious spreadsheet management.
In addition, when MDM and IS teams impose very structured processes to create and purchase materials, that structure can put more of a burden on maintenance staff, for example, as when requesting repair work orders. To meet those work order deadlines, they may opt to use a procurement card instead, which only contributes to the problem.
External Consulting and Cleansing Costs
Alternatively, companies can hire external consulting firms that often rely on offshore resources to clean data and provide data exports. Their teams spend cycles manually checking the data and then cleaning it up for export back into the company’s ERP or other inventory management system—a process that can take months. The real issue with this approach is that once the cleansed data is uploaded back to the system, it can quickly become dirty again if no controls are put into place to avoid bad data habits, such as inconsistent part naming across the organization.
The other downside of the external approach is cost. Because data cleansing is a complex, multi-step process that requires a specialized set of software, people and procedures,⁹ the costs add up. Depending on what is included, the typical cost for data cleansing for a database of 10,000 records ranges from $5,000 to $15,000, with costs broken down as follows¹⁰:
Costs Associated with Data Cleansing Tactics
- Cost of removing duplicates – To de-dupe 10,000 records would cost $1,000 to $3,000, depending on whether an automatic de-duping tool is used or a de-duplication service provider.
- Cost of appending data – Appending missing data can cost from $0.50 to $5.00 per record, depending on data type and source. So, if 30 percent of a database is missing at least one field, it costs from $1,500 to $15,000.
- Cost of bad data – Validating and cleaning bad records costs $0.05 to a $1.00 depending on the data provider and what is being verified, so for 10,000 records that cost equals $500 to $10,000.
AI Emerges as an Industry 4.0 Answer for Inventory Management
According to Deloitte, Industry 4.0’s “ability to adjust to and learn from data in real time can make organizations more responsive, proactive and predictive, and enables the organization to possibly avoid operational downtime and other productivity challenges.”¹¹ More specifically, AI is emerging as an Industry 4.0 technology that can be applied to address materials inventory and data management challenges, keeping procurement in check and production running smoothly for supply chain efficiency. In short, AI is creating new, dynamic possibilities for materials inventory data management.
Here are some of the ways AI meets the value proposition when applied in materials inventory management:
Harmonizes Disparate Inventory Systems Data: AI-based solutions automatically integrate with ERP and disparate data sources from multiple systems or locations. Then, AI kicks in to learn from your company’s inventory experts, encoding this knowledge to provide data harmonization and a true, accurate materials master so that uptime and supply chain efficiency are preserved. By automating data harmonization with AI, continuous improvement is possible.
Controls Policy and Procedures for Proactive Materials Management: When disparate inventory data is harmonized, employees both search for the right materials and see their true quantities—across the enterprise and not in local silos—to prevent over-purchasing.
Predicts Inventory Needs for Continuous Improvement: AI builds on the knowledge of your inventory experts to create continuous application and improvement, including offering suggestions to continually optimize inventory allocation and procurement needs. Inventory reports keep your teams performing at the highest level while building trust through accuracy between experts and the harmonized data.
Finally, AI returns a measure of control to employees by providing valuable insights into the ways that databases actually work, allowing them to learn from past mistakes to build better information for the future. It is truly an Industry 4.0 technology that “can make organizations more responsive, proactive and predictive.”
AI Accelerates Inventory Reduction for Fortune 500 Manufacturer
AI is already making its name in the manufacturing sector. For example, consider the case of a Fortune 500 pulp and paper manufacturer with more than 60 North American facilities.
After several acquisitions, the company’s chief supply chain officer (CSCO) introduced an aggressive initiative to reduce working capital by $5 million, obtain enterprise inventory visibility and rebalance inventory through a virtual maintenance, repair and operating (MRO) inventory network. When the team began to execute the MRO spare parts inventory optimization plan, it quickly became apparent that there was a lack of visibility across the company’s multiple systems that contained material master (MM) data, including SAP, as well as inconsistent data quality. After assessing the scope of the necessary data cleanse, the team estimated it would fall at least one year behind its inventory reduction targets if employing only traditional cleansing methods and technologies. As a promising alternative from MRO spare parts optimization examples, the team turned to an AI-enabled platform.
With the AI-enabled platform, the team was able to lay the appropriate data foundation by structuring its MM data in weeks instead of a full year. The AI and machine learning platform automated the material entry process and harmonized the inventory data across all MM catalogs. It also provided an accurate, trustworthy view of enterprise MRO inventory, as required. The AI-enabled platform ultimately cleansed the MRO software data, allowing the company to begin a “self-cleansing” data strategy, as well as an internal “buy-from-self-first” inventory optimization strategy and solution. Additionally, once the data was cleansed, the platform delivered optimized insights on where inventory could be reduced as well as how to avoid creating additional excess.
In just two months, the AI-enabled platform identified the $5 million goal for reduction in working inventory. Although the platform revealed that the company’s inventory problem was worse than estimated, its fast results and visibility into what items were truly overstocked encouraged the CSCO to raise the MRO savings target from $5 million to $20 million for the same time period as the original plan. The MRO inventory management software and platform continue to learn from the company’s inventory activities, execute data cleansing and provide scaled inventory optimization software insights and opportunities.
Predictions for the adoption of AI to improve inventory and supply chain processes like it did in this large company are extremely favorable. According to Gartner, by 2023, at least 50 percent of large global companies will be using AI, advanced analytics and IoT in supply chain operations.¹²
The Future is Now: AI, Industry 4.0 and Business Agility
In today’s business climate, companies can’t afford to trust manual processes and data stewardship while achieving the speed and scale necessary to compete in dynamic markets. The more aggressively an organization is looking to digitize and embrace Industry 4.0, the more important having a trusted data foundation and underlying intelligence become. According to Deloitte, smart, connected Industry 4.0 technologies like AI “can transform organizations themselves: how they make sense of information and act upon it to achieve operational excellence and continually improve the consumer/partner experience.”¹¹
AI’s application in materials inventory and data management will result in systems that are more usable, more responsive and more capable than ever to support agile supply chains. The emergence of AI is replacing frustration with the ability to better leverage data for more strategic, creative opportunities to propel supply chains forward. Bad data is costly, but AI can easily and cost-effectively restore the value of building and maintaining accurate and accessible inventory data.
Endnotes
- Banker, Steve, “The Costs of Excess Inventory Can Be Huge,” Forbes, 10 March 2016.
- Schwab, Klaus, The Fourth Industrial Revolution, Penguin Books Ltd., 2017.
- Marr, Bernard, “What is Industry 4.0? Here’s a Super Easy Explanation for Anyone,” Forbes, 2 September 2018.
- Asthana, Rahul, “Making Sense of Supply Chain 4.0,” Industry Week, 2 November 2018.
- de Baat, Bas, “Artificial Intelligence is transforming ERP solutions,” CIO, 17 October 2016.
- Advanced Technology Services/Nielsen Research, “Downtime Costs Auto Industry $22K/Minute-Survey,” Advanced Technology Services, 27 March 2005.
- SDCExec.com, “Survey Reveals Performance Gap Procurement: LevaData’s annual survey on Strategic Sourcing and Procurement uncovers widening performance gap,” Supply & Demand Chain Executive, 29 October 2018.
- Umbenhauer, Brian and Younger, Lance, Leadership: Driving innovation and delivering impact | The Deloitte Global Chief Procurement Officer Survey 2018, Deloitte Development LLC, 2018.
- Facciotti, Joceyln, “Reduce Costs with MRO Data Cleansing,” Reliable Plant, n.d.
- StrategicDB, “The True Cost of Data Cleaning,” StrategicDB Corp., 18 December 2017.
- Cotteleer, Mark and Sniderman, Brenna, “Forces of change: Industry 4.0,” Deloitte Insights, 18 December 2017.
- Panetta, Kasey, “Supply chain leaders must examine how emerging technologies such as AI, robotics and blockchain will affect their business, and decide where and how to invest,” Smarter with Gartner, 17 December 2018.